Multi tenancy task scheduler
Last time I showed how to extend Spring default request handler adapter so that we are able to schedule or reject incoming requests. The goal of the TenantTaskCoordinator
is to:
- queue requests for processing
- limit the maximum number of concurrently processed requests
- reject requests after the maximum queue size is reached
- interrupt processing of a request upon an upstream subscription disposal
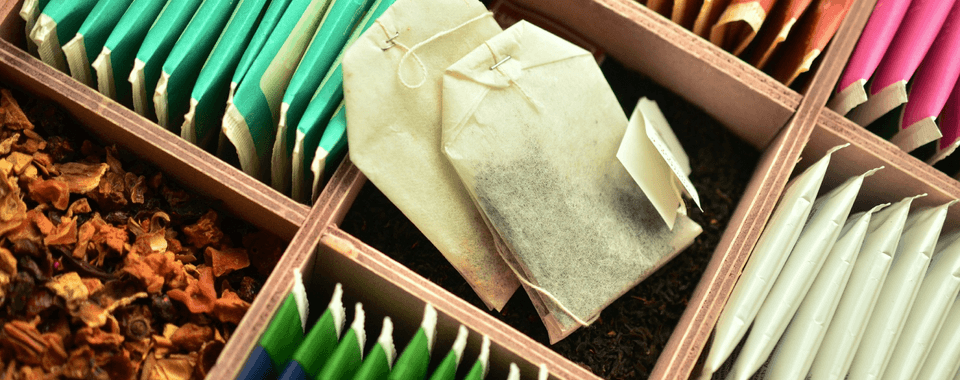
Tenant task coordinator execute method
Our entry point into TenantTaskCoordinator
is a single method fun <T : Any> execute(job: Callable<T>): Mono<T>
:
fun <T : Any> execute(job: Callable<T>): Mono<T> {
return Mono.create({ outsideSink ->
val _workInProgressWasDecremented = AtomicBoolean(false)
fun decrementOnce() {
if (_workInProgressWasDecremented.compareAndSet(false, true)) {
currentWorkInProgressCounter.decrementAndGet()
}
}
val workInProgress = currentWorkInProgressCounter.incrementAndGet()
if (workInProgress > maximumWorkInProgress) {
outsideSink.error(TooManyTasks("Work in progress $workInProgress exceeds $maximumWorkInProgress jobs in $name"))
decrementOnce()
} else {
val singleJob = Mono.fromCallable(job).doAfterTerminate {
decrementOnce()
}
val delayedTask = Task(name, singleJob as Mono<Any>, outsideSink as MonoSink<Any>)
outsideSink.onCancel {
delayedTask.outsideCancel()
decrementOnce()
}
taskSink.next(delayedTask)
}
})
}
The first step is to return Mono<T>
which is simply done with Mono.create
. The sink
we get passed is used to control the outcome observed from outside. It also allows for registering an onCancel
callback invoked when the upstream cancels its subscription.
The _workInProgressWasDecremented
is used to guard and decrement the currentWorkInProgressCounter
in a thread safe fashion. We first check whether we have immediately exceeded the maximum number of queued jobs. If the threshold is reached, we notify the observer about the error with outsideSink.error
.
If we have enough capacity to a perform job
, we convert it to a reactive world with Mono.fromCallable
and attach a doAfterTerminate
callback that decrements the work in progress counter. The Task
class links singleJob
and outsideSink
so that they are both accessible while processing. Finally, we schedule the task
through taskSink.next(delayedTask)
.
Task coordinator state
Let's have a look at the task coordinator state variables and how they are initialized:
class TenantTaskCoordinator(private val scheduler: Scheduler,
val maximumConcurrency: Int = 1,
val maximumQueueSize: Int = 50,
val name: String = "") : AutoCloseable {
private val maximumWorkInProgress = maximumQueueSize + maximumConcurrency
private val maxBufferSize = maximumWorkInProgress * 2
val currentWorkInProgressCounter = AtomicInteger()
private lateinit var taskSink: FluxSink<Task>
private val taskSource = Flux.create<Task>({ taskSink = it }, FluxSink.OverflowStrategy.BUFFER)
private val processSinkOnErrorResume = processSinkWithLimitedConcurrency()
.onErrorResume { error: Throwable? ->
LOG.warn("name={} Error processing sink with limited concurrency", name, error)
processSinkWithLimitedConcurrency()
}
The first interesting part is how we setup taskSink
by using Flux.create
. For clarity, we explicitly pass FluxSink.OverflowStrategy.BUFFER
so that tasks are buffered in case they outpace the processor. The name
is used to get better log messages. Finally, we call processSinkWithLimitedConcurrency
to start task processing using the given scheduler
. Interestingly the onErrorResume
restarts the processing in case we have a bug.
Task coordinator concurrent processing
The most important and tricky to figure out part is to correctly process jobs. It took me several back and forth steps until I got the order of reactive API calls right.
private fun processSinkWithLimitedConcurrency(): Flux<Any> {
return taskSource
.filter { !it.isCancelled }
.flatMap({ task ->
task.work
.doOnError(task::onError)
.doOnSuccess(task::onSuccess)
.subscribeOn(scheduler)
.timeout(task.outsideTimeout)
.onErrorReturn(task)
}, maximumConcurrency, maxBufferSize)
}
First, we filter out tasks that are already cancelled. Then, we use flatMap
overload to process tasks with given maximum concurrency. The flatMap
callback delegates most of the work to the mentioned Task
instance. The onErrorReturn
effectively suppresses any errors that might occur during task
execution. Let's see how the inner Task
class looks like:
private data class Task(val name: String,
private val job: Mono<Any>,
val outsideSink: MonoSink<Any>,
@field:Volatile var isCancelled: Boolean = false) {
val work: Mono<Any> get() = if (isCancelled) Mono.empty() else job
lateinit var outsideTimeoutSink: MonoSink<Task>
val outsideTimeout = Mono.create<Task> { outsideTimeoutSink = it }
fun outsideCancel() {
isCancelled = true
outsideTimeoutSink.success(this)
}
fun onSuccess(result: Any?) {
outsideSink.success(result)
}
fun onError(error: Throwable) {
LOG.warn("Task.onError {}", this, error)
outsideSink.error(error)
}
}
The job
argument is the Callable
passed to the execute
method. The outsideTimeout
signals when the task
instance subscription is cancelled. The signal is propagated inside processSinkWithLimitedConcurrency
with a Mono.timeout
call and breaks the task
processing. Last but not least the onSuccess
and onError
simply push the result or error to the outsideSink
effectively notifying the observer of the result.
The TenantTaskCoordinator
was not simple to figure out given the requirements mentioned at the begging of the post. I'm pleased with the final result although I must say it was not intuitive to figure out how to combine all the nuts and bolts of Reactor library to achieve the desired outcome.